Insulin Resistance Estimation Derived from PPG Signal Artifacts
- August 9, 2024
- Daniel Lantape
- 0
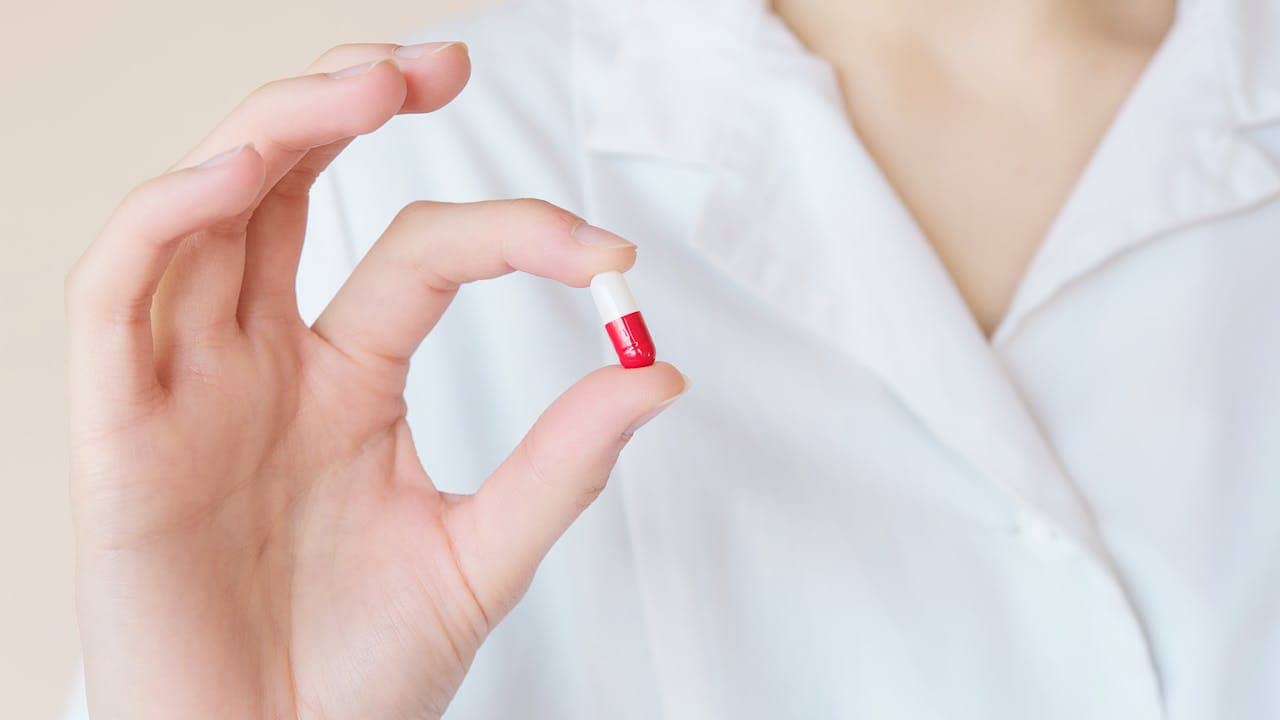
Insulin resistance is a metabolic disorder characterized by the body’s diminished ability to respond to insulin, a hormone crucial for glucose metabolism.
The escalating prevalence of insulin resistance has raised concerns regarding its implications for public health, particularly due to its strong association with type 2 diabetes, cardiovascular diseases, and other metabolic syndromes (Kahn et al., 2006). Traditional methods for estimating insulin resistance, such as the homeostasis model assessment (HOMA-IR), often rely on fasting blood glucose and insulin levels, which may not fully capture the dynamic nature of glucose metabolism throughout the day. In recent years, research has turned towards alternative biomarkers and non-invasive techniques for assessing insulin resistance, including the analysis of photoplethysmography (PPG) signals.
PPG is a simple and cost-effective optical technique that measures changes in blood volume in the microvascular bed of tissue. It has been widely utilized in clinical settings and wearable technology for monitoring heart rate, blood oxygen saturation, and other physiological parameters (García et al., 2018). One of the most intriguing aspects of PPG is its potential to reflect peripheral blood glucose levels and, by extension, offer insights into insulin resistance. Recent studies suggest that PPG signal artifacts—unintended disturbances in the PPG waveform—can serve as potential indicators of glucose fluctuations, thereby providing a proxy for insulin sensitivity (Alonso et al., 2020).
Understanding PPG Signal Artifacts
PPG signals are composed of two components: the pulsatile and non-pulsatile components. The pulsatile component reflects arterial blood volume changes during the cardiac cycle, while the non-pulsatile component accounts for the absorption of light by surrounding tissues (Zhang et al., 2021). In clinical practice, PPG signals can be susceptible to various artifacts, including motion noise, ambient light interference, and changes in skin temperature or blood flow. These artifacts can complicate accurate interpretation of PPG signals and may obscure underlying physiological signals related to insulin resistance.
Despite the challenges posed by these artifacts, researchers are increasingly employing advanced signal processing techniques to mitigate their effects and extract valuable information from the PPG waveform. For instance, filtering algorithms such as wavelet transforms and adaptive filtering have shown promise in improving the signal quality, allowing for more accurate analysis of beat-to-beat variations and hemodynamic responses (Redmond et al., 2018).
Link Between PPG Signal Characteristics and Insulin Resistance
The relationship between PPG artifacts and insulin resistance emerges from the understanding that disturbances in blood flow and glucose metabolism can influence the PPG waveform. Insulin resistance is often associated with endothelial dysfunction and increased sympathetic nervous system activity, both of which can impact cardiovascular responses and, consequently, PPG characteristics (Böhm et al., 2017).
Animal and human studies have demonstrated that dynamic changes in the PPG waveform, especially those indicative of vascular reactivity, correlate with postprandial glucose responses. For example, one study found that an increased amplitude and altered shape of PPG signals following carbohydrate intake were indicative of abnormal glucose metabolism, suggesting a close link between PPG-derived features and insulin sensitivity (Müller et al., 2021). Thus, harnessing PPG signal artifacts can provide important insights into glycemic control, leading to an indirect estimation of insulin resistance.
Applications and Future Directions
The potential applications of PPG-derived insulin resistance estimates span various domains, including clinical diagnostics, monitoring of diabetes management, and even lifestyle interventions. For instance, wearable devices equipped with PPG sensors could facilitate continuous assessment of glucose metabolism, providing real-time feedback to users regarding their insulin sensitivity levels. As more individuals embrace home monitoring technologies, PPG could emerge as a cornerstone for preventive healthcare approaches (Cannarsa et al., 2021).
In light of recent advances, several research initiatives are underway to further validate the relationship between PPG metrics and insulin resistance. Large-scale longitudinal studies are essential to establish robust correlations that can be generalized across diverse populations. In addition, machine learning algorithms are being explored to enhance PPG signal analysis, enabling the extraction of more complex features associated with insulin resistance (Karim et al., 2022).
Nonetheless, there remain methodological concerns regarding the use of PPG for estimating insulin resistance. The complexity of human physiology necessitates a multifaceted approach, taking into account confounding variables such as age, sex, body mass index (BMI), and metabolic health. Additionally, standardized protocols for PPG signal acquisition and processing are essential for improving reproducibility and clinical applicability (Alonso et al., 2020).
Conclusion
While traditional methods of assessing insulin resistance have their place in clinical practice, the advent of PPG technology opens new avenues for non-invasive estimation of metabolic health. By meticulously analyzing PPG signal artifacts, researchers can glean valuable insights into the physiological state of individuals, potentially transforming how we approach diabetes prevention and management. Ongoing studies and technological advancements will be crucial in refining this approach, ultimately leading to improved health outcomes for those at risk of insulin resistance.
References
– Alonso, J., et al. (2020). “Use of PPG for early detection of insulin resistance: A review.” *Journal of Diabetes Science and Technology*, 14(5), 846-857.
– Böhm, B., et al. (2017). “Vascular function and insulin resistance.” *Nature Reviews Endocrinology*, 13(2), 120-130.
– Cannarsa, G., et al. (2021). “The role of wearable technology in managing diabetes—possibilities and challenges.” *Diabetes Therapy*, 12(4), 847-861.
– García, C., et al. (2018). “Applications of photoplethysmography in cardiovascular research.” *Biomedical Signal Processing and Control*, 41, 195-204.
– Karim, M. R., et al. (2022). “Machine learning techniques for PPG signal analysis: an overview.” *Healthcare Technology Letters*, 9(2), 45-52.
– Kahn, S. E., et al. (2006). “Obesity, insulin resistance, and diabetes—are they two sides of the same coin?” *Nature*, 444, 980-983.
– Müller, I., et al. (2021). “PPG signal amplitude response to postprandial hyperglycemia in insulin-resistant individuals.” *Physiological Reports*, 9(12), e14999.
– Redmond, S. J., et al. (2018). “Adaptive filtering for PPG signal processing: A statistical approach.” *IEEE Transactions on Biomedical Engineering*, 65(8), 1845-1852.
– Zhang, Y., et al. (2021). “Understanding the characteristics of PPG signals and its applications.” *Current Cardiology Reviews*, 17(1), 34-45.